Duuk Baten
Digitale innovatie voor onderwijs en onderzoek. Nu ben ik binnen SURF bezig… Meer over Duuk Baten
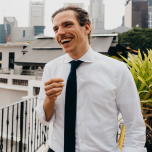
In the ever-evolving realm of scientific research, the advent of Artificial Intelligence (AI) has been like the discovery of a new frontier. Yet, it isn't without its hurdles. This was a central topic during our panel session at SURF Research Day 2023, where we delved into the intricacies of employing machine learning as a research methodology.
Science history brims with instances where initial observations or conceptions have been misleading. In the 17th-century, astronomers mistook the moon's dark spots for seas, dubbing them as 'maria,' based on an assumption that, like Earth, the moon also harbored seas. These 'seas' were later found to be ancient lava plains. More recently, the quantum mechanics revolution challenged the classical mechanics' determinism, turning our understanding of the world on its head. Such instances remind us that scientific pursuit is a journey - often of correcting our own misconceptions.
In a panel discussion with our five excellent panelists, we engaged in a deep conversation about the philosophical roots of AI in research, focusing on the epistemology of machine learning. The term 'epistemology,' derived from the Greek words 'epistēmē' (knowledge) and 'logos' (reason), refers to the philosophical investigation of human knowledge—a crucial aspect of scientific inquiry. With the increasing use of AI in science, we thought it was interesting to explore this complex relation between the two. As stated in a still to be published OECD report on scientific and technological policy, "Accelerating the productivity of research could be the most economically and socially valuable of all the uses of artificial intelligence (AI)." But, the marriage of AI and the traditional 'scientific method' raises some thought-provoking questions.
The panel:
Our discussion covered questions like 'What constitutes scientific knowledge?' and 'How do machine learning models align with the hypothesis-testing procedure in science?' We analyzed research in the philosophy of science, differentiating between human and machine epistemology as they often diverge. We also discussed the practical application of machine learning in science, addressing challenges such as determining uncertainty levels in model results, and the need for ML models to build upon existing knowledge, not just “stirring in the data pot”.
From this rich dialogue, several insights emerged:
AI and machine learning are impacting scientific research. As our panel discussion highlighted, we need to be careful navigators of this new terrain. I want to thank our panelists for the great conversation, our participants for their attention and their questions, and my colleague Yue Zhao for co-organizing this.
If you are working with machine learning in your research, we are curious to learn more about your way of working. Please take a moment to help us and fill in our short (10 min) survey about responsible AI practices in research. We have launched this survey to get a better insight in the use of AI or machine learning within research and the accompanying tools, policies, and practices. All of this as an attempt to better understand what the role of SURF in this domain can and/or should be.
Digitale innovatie voor onderwijs en onderzoek. Nu ben ik binnen SURF bezig… Meer over Duuk Baten
0 Praat mee