Nynke Kruiderink
Ik werk sinds 1 December 2021 in het kernteam innovatie bij SURF. Een hele… Meer over Nynke Kruiderink
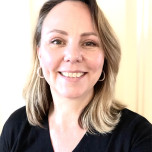
I read a recent interview with John Hattie wherein he expresses one of the greatest frustrations of the Learning Sciences is identifying successful teaching and then struggling to scale it up. As a company by and for all HigherEd institutions in the Netherlands, SURF aims to focus on delivering services which are scalable. Within the field of Learning Analytics this challenge increases incrementally the closer you get to classroom activities as teachers have so much autonomy in their course design and there is no one size fits all for learning. But SURF wouldn’t be SURF if we didn’t challenge ourselves to push the boundary as far as we can and explore the edges of the possibilities together with our members.
This is the aim in the Project Experimenting within the Programme Educational Data and AI, to explore how we can implement Learning Analytics at scale, and what SURFs role can be in facilitating this. Last year we explored this topic together with eleven educational institutions during interactive sessions together with an IT architect from SURF, project leader and advisor. As SURF we spoke to many educational institutions about their data architecture, data lakes and record stores, but we also explored different international examples in educational data infrastructures such as those deployed by JISC.
In our exploration the workgroup members collaboratively formulated a concept, a shared ambition in the form of a user case which was a Student Facing Dashboard. These dashboards are intended to provide students with data and insights about their learning progress, helping them to identify areas of strength and weakness, set goals, and make informed decisions about their academic path. They are visual representations of learning traces combined with other data such as grades, wellbeing, attendance and possibly comparisons with peers.
While Student Facing Dashboards have the potential to provide valuable insights, they are not without potential drawbacks. Firstly, Student Facing Dashboards may not be effective for all students. Some students may not find the information presented on the dashboard useful or may struggle to interpret the data. Additionally, research has shown that some students may feel overwhelmed or stressed by the constant monitoring of their academic progress, leading to increased anxiety and a decrease in motivation (Gasevic, Dawson, & Siemens, 2015).
Furthermore, Student Facing Dashboards may perpetuate the focus on grades as the sole measure of academic success. This narrow focus may not account for other important factors that contribute to student learning, such as student engagement and self-efficacy (Baker, 2016). As such, it is important to consider the limitations of grades as a measure of academic success and to incorporate a more holistic approach to Learning Analytics.
Additionally, Student Facing Dashboards may also present issues of privacy and surveillance. Some students may feel uncomfortable with the amount of data being collected and monitored by their institution, especially if they are not fully aware of how this data is being used. Furthermore, students from marginalized backgrounds may be disproportionately impacted by surveillance measures, as they may already be subject to increased scrutiny and surveillance (Gupta, 2017).
Being aware of these issues is very important when moving forward with the selected use case. In Learning Analytics there are always many issues to consider and never a one size which fit all. But we can rise to the challenges student dashboards face us with. As John F. Kennedy said, we go to the moon because it is hard.
For example regarding privacy and surveillance issues, it is precisely through developing Student Facing Dashboards that we can foster new conversations within educational institutions with students about the data which is being gathered about them, and how they would like this data to be used. The recent exploration SURF conducted on Control on Studiedata by Students demonstrates that there is a dire need to engage in these conversations. (https://www.surf.nl/verkenning-regie-op-studiedata-door-studenten )
Furthermore, the argument that Student Facing Dashboards may focus too much attention on grades alone, is mute in our case because the dashboard the workgroup designed also included student wellbeing as an important component. At this time, we are not always recording that data, so it would be difficult to include it in a generic dashboard, but theoretically the workgroup is certainly in favor of a wholistic approach to Learning Analytics and the Student Facing Dashboard.
Finally, that a standard Student Facing Dashboard may not be effective for all students and even may be detrimental for some, is part of the frustration I think Hattie was referring to. There is not one magic button for all learners (or teachers) to push which will increase learning in an effective manner. But if we are scoping the future and exploring dashboards which could possibly work at scale, then there will probably be a lot of personalization involved. Either by teachers, by students themselves, or even in an automated fashion with the use of AI. Personalization in a dashboard is of course very achievable with standard widgets to pick and choose from or suggested to you based on your demonstrated personality traits which may influence your learning behavior. This is a field of research which is also growing and continuing to provide us with new insights on how to personalize content for our learners as I recently learned about during my visit to the annual Online Educa Conference in Berlin, see the “Predicting culture and personality in online courses” .
The first phase of the experimentation has been completed. We will now proceed with a smaller group of institutions who are ready to execute a Proof of Concept (POC). While developing a shared Student Facing Dashboard together brings plenty of challenges it also allows us to explore and engage in new conversations and also for SURF to experiment with facilitating data input from different sources and processing this in a manner which allows the educational institution to apply it so they can further increase the quality of education offered at the institution.
Ahmed Tlili, Rim Fadhelaoui, Marwa Zaghdoudi, Nian-Shing Chen, Kinshuk (2019). Predicting culture and personality in online courses. Conference: Proceedings of the SLLL 2019: Workshop on Supporting Lifelong Learning, Held in conjunction with the 20th International Conference on Artificial Intelligence in Education, AIED2019. June 25th – June 29th 2019, Chicago, USA.
Baker, R. S. (2016). Early warning systems: Mining students’ online behaviors for dropout prediction. IEEE Transactions on Learning Technologies, 9(1), 5-13.
Gasevic, D., Dawson, S., & Siemens, G. (2015). Let’s not forget: Learning analytics are about learning. TechTrends, 59(1), 64-71.
Gupta, M. (2017). Privacy, surveillance, and power: Understanding the ‘data-driven’ state. Television & New Media, 18(7), 591-607.
===================================================
Recentelijk las ik een interview met John Hattie waarin hij een van de grootste frustraties van de leerwetenschappen uitdrukt, namelijk het identificeren van succesvol onderwijs en het opschalen daarvan. Als bedrijf dat door en voor alle Hoger Onderwijsinstellingen in Nederland is opgericht, streeft SURF ernaar om zich te richten op het leveren van schaalbare diensten. Binnen het veld van Learning Analytics neemt deze uitdaging echter toe naarmate je dichter bij de activiteiten in de klas komt, omdat leraren zoveel autonomie hebben in hun cursusontwerp. Maar SURF zou niet SURF zijn als we onszelf niet zouden uitdagen om de grens zo ver mogelijk te verleggen en samen met onze leden de mogelijkheden te verkennen.
Dit is het doel van het project "Experimenteeromgeving voor Learning Analytics ", om te verkennen hoe we Learning Analytics op schaal kunnen implementeren en wat de rol van SURF kan zijn bij het faciliteren hiervan. Vorig jaar hebben we dit onderwerp samen met elf onderwijsinstellingen verkend tijdens interactieve sessies samen met een IT-architect van SURF, projectleider en adviseurs. Als SURF hebben we gesproken met veel onderwijsinstellingen over hun data-architectuur, data lakes en -opslag, maar we hebben ook verschillende internationale voorbeelden verkend van educatieve data-infrastructuren, zoals die van JISC.
Tijdens onze verkenning formuleerden de leden van de werkgroep gezamenlijk een concept, een gedeelde ambitie in de vorm van een use-case, namelijk een Student Facing Dashboard. Deze dashboards zijn bedoeld om studenten gegevens en inzichten te bieden over hun leerproces, waardoor ze sterke en zwakke punten kunnen identificeren, doelen kunnen stellen en beslissingen kunnen nemen op basis van deze informatie over hun leerpad. Het zijn visuele representaties van leertraces gecombineerd met andere gegevens zoals cijfers, aanwezigheid en mogelijke vergelijkingen met medestudenten.
Hoewel Student Facing Dashboards waardevolle inzichten kunnen bieden, hebben ze ook potentiële nadelen. Ten eerste zijn Student Facing Dashboards mogelijk niet effectief voor alle studenten. Sommige studenten vinden de gepresenteerde informatie op het dashboard mogelijk niet nuttig of hebben moeite om de gegevens te interpreteren. Bovendien heeft onderzoek aangetoond dat sommige studenten zich overweldigd of gestrest kunnen voelen door de constante monitoring van hun voortgang, wat kan leiden tot verhoogde angst en een afname van motivatie (Gasevic, Dawson, & Siemens, 2015).
Bovendien kunnen Student Facing Dashboards de focus op cijfers als enige maatstaf voor studiesucces in stand houden. Deze smalle focus houdt mogelijk geen rekening met andere belangrijke factoren die bijdragen aan het leerproces van studenten, zoals betrokkenheid en ‘self-efficacy’ van studenten (Baker, 2016). Het is daarom belangrijk om de beperkingen van cijfers als maatstaf voor studiesucces te overwegen en een meer holistische benadering van Learning Analytics te integreren.
Bovendien kunnen dashboards voor studenten ook privacy- en surveillanceproblemen opleveren. Sommige studenten kunnen zich ongemakkelijk voelen bij de hoeveelheid gegevens die door hun instelling worden verzameld en gemonitord, vooral als ze zich niet volledig bewust zijn van hoe deze gegevens worden gebruikt. Bovendien kunnen studenten uit achtergestelde groepen onevenredig worden getroffen door surveillancemaatregelen, omdat zij mogelijk al onderhevig zijn aan verhoogd toezicht en surveillance (Gupta, 2017).
Het is belangrijk om rekening te houden met deze kwesties bij het voortzetten van het gebruik van studentdata. Bij Learning Analytics zijn er altijd veel kwesties om te overwegen en er is nooit een oplossing die voor iedereen geschikt is. Maar we kunnen de uitdagingen van studentendashboards samen aangaan.
Bijvoorbeeld met betrekking tot privacy- en surveillancekwesties kunnen we juist door het ontwikkelen van studentendashboards nieuwe gesprekken op gang brengen binnen onderwijsinstellingen met studenten over de gegevens die over hen worden verzameld en hoe ze willen dat deze gegevens worden gebruikt. De recente verkenning van SURF naar controle over studiegegevens door studenten laat zien dat er een grote behoefte is om deze gesprekken te voeren. (https://www.surf.nl/verkenning-regie-op-studiedata-door-studenten)
Bovendien is het argument dat studentendashboards te veel nadruk leggen op alleen cijfers alleen, irrelevant in ons geval omdat het dashboard dat de werkgroep heeft ontworpen ook het welzijn van studenten als een belangrijk onderdeel bevat. Op dit moment registreren we die gegevens niet altijd, dus het zou moeilijk zijn om ze op te nemen in een generiek dashboard, maar theoretisch staat de werkgroep zeker open voor een holistische benadering van Learning Analytics en het studentendashboard.
Ten slotte kan een standaard studentendashboard niet effectief zijn voor alle studenten en zelfs schadelijk zijn voor sommigen, en dat is volgens mij waar Hattie op doelde. Er is geen magische knop voor alle studenten (of docenten) die het leren op een effectieve manier verhoogt. Maar als we de toekomst verkennen en dashboards onderzoeken die mogelijk op grote schaal kunnen werken, zal er waarschijnlijk veel personalisatie mee gemoeid zijn. Ofwel door docenten, door de studenten zelf, of zelfs automatisch met behulp van AI. Personalisatie in een dashboard is natuurlijk heel goed haalbaar met standaard widgets om uit te kiezen of die worden voorgesteld op basis van uw persoonlijkheidseigenschappen die het leer- en gedragspatronen beïnvloeden. Dit is een groeiend onderzoeksgebied dat ons blijft voorzien van nieuwe inzichten over hoe we inhoud kunnen personaliseren voor onze leerlingen, zoals ik onlangs tijdens mijn bezoek aan de jaarlijkse Online Educa-conferentie in Berlijn heb geleerd.
De eerste fase van het experiment is afgerond. We zullen nu doorgaan met een kleinere groep instellingen die klaar zijn om een Proof of Concept uit te voeren. Het ontwikkelen van een gedeeld, student-facing dashboard brengt veel uitdagingen met zich mee, maar het stelt ons ook instaat om nieuwe gesprekken te verkennen. Daarnaast is dit voor SURF de kans om te experimenteren met het faciliteren van een omgeving, waarbij data vanuit verschillende bronnen van een onderwijsinstelling verwerkt wordt. Dit alles met als grote doel om de kwaliteit van het onderwijs verder te verhogen.
Ik werk sinds 1 December 2021 in het kernteam innovatie bij SURF. Een hele… Meer over Nynke Kruiderink
0 Praat mee