Duuk Baten
Digitale innovatie voor onderwijs en onderzoek. Nu ben ik binnen SURF bezig… Meer over Duuk Baten
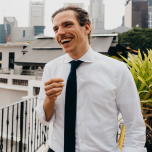
OpenAI has launched a new service, ChatGPT that combines the power of its language model GPT-3 with the ease of use of a chatbot interface. The AI community is buzzing with this new development, but what does this mean for education? With the increasing abilities and availability of language models, it becomes hard to judge text on face-value. This is a trend we already mentioned in SURF’s recent Promises of AI in Education report [1]. So, what does this mean for education? Are these systems good enough to pass standard academic assessment?
When even the Volkskrant publishes an article on the development of a new AI-driven application, you know something interesting is happening[2]. The impact of language models on education has been on my mind for a while, but like a lot of people this recent development shocked me with its capabilities and ease of use. So what is happening? OpenAI, the company behind GPT-3 and DALL-E (two of the most impressive AI developments this year), has launched a new service called ChatGPT which combines the power of GPT-3 with the ease of use of a chatbot interface. You can basically ask it a question (a prompt) and then the model returns with a response. A response that can be remarkably convincing.
To discuss the impact of ChatGPT for a moment I flirted with the idea ask the model to write and edit this whole blog. A trick that would show ChatGPTs potential to generate convincing text but would leave open the more interesting questions. So in this blogpost I will use elements of text produced by ChatGPT together with the prompt and discuss those to get an insight into what these systems can do. Let’s take a look at one.
Prompt: “Currently language models such as GPT-3 and ChatGPT are rapidly increasing in abilities. This can be a worrying trend for educational institutions, that still depend quite heavily on essay-based assessment. Write a blogpost with headings and references about how these language models work and what there[3] impact on education will be.”
On the first attempt:
The Impact of Language Models on Education
Language models, such as GPT-3 and ChatGPT, are rapidly advancing in their ability to understand and generate human-like text. This can be a concern for educational institutions that rely heavily on essay-based assessment, as it raises questions about the reliability of such assessments in the face of increasingly sophisticated language generation technology. In this blog post, we will explore how these language models work and discuss their potential impact on education.How Language Models Work
Language models are machine learning algorithms that are trained on large datasets of text. The goal of these models is to predict the next word in a sequence of words based on the words that came before it. This allows them to generate coherent and grammatically correct sentences and paragraphs.
One of the key challenges in training language models is the sheer amount of data that is required. The larger the dataset, the more accurately the model can learn the nuances of human language. This has led to the development of extremely large language models, such as GPT-3, which is trained on a dataset of over 40 billion words.The Impact of Language Models on Education
As language models become more sophisticated, they have the potential to impact the way that essays and other written assignments are assessed in education. For example, it may become possible for a student to simply input a prompt into a language model and receive a well-written and coherent essay in response.This could potentially make traditional forms of essay-based assessment less effective, as it would be difficult for educators to distinguish between essays written by a student and those generated by a language model. It could also create a situation where students with access to these language models have an unfair advantage over those who do not.
One possible solution to this problem would be to use other forms of assessment that are not as susceptible to manipulation by language models. For example, educators could focus on assessing students' critical thinking skills through problem-solving assignments and projects, rather than relying on essays as the primary means of evaluation.Conclusion
Language models are a powerful tool for generating human-like text, but their increasing sophistication raises concerns for the reliability of essay-based assessment in education. As these models continue to advance, it will be important for educators to consider alternative forms of assessment that are not as susceptible to manipulation by language generation technology.
So within about 30 seconds of work, this would be an impressive result. And it could easily do the same in Dutch as well. The generated paragraphs read well and seem consistent but miss perhaps some aspects that are needed to pass the academic rigor required in most educational programs. For example, references to background literature.
Let’s try to fix that with the following prompt “Can you further specify the section 'How language models work' into how the models are trained and how the models generate the text and include inline academic references following APA reference style.”[4].
How Language Models Work
Language models are machine learning algorithms that are trained on large datasets of text. The goal of these models is to predict the next word in a sequence of words based on the words that came before it (Bengio, Courville, & Vincent, 2015). This allows them to generate coherent and grammatically correct sentences and paragraphs.Training a language model involves feeding it a large dataset of text and using algorithms to adjust the model's internal parameters until it can accurately predict the next word in a sequence (Radford et al., 2019). This process is known as supervised learning, as the model is "supervised" by the labeled training data.
Once the model has been trained, it can be used to generate text by inputting a prompt and iteratively predicting the next word in the sequence, using the previous words as context (Vaswani et al., 2017). This allows the model to generate text that is coherent and grammatically correct, but also maintains the style and tone of the original prompt.
One of the key challenges in training language models is the sheer amount of data that is required. The larger the dataset, the more accurately the model can learn the nuances of human language. This has led to the development of extremely large language models, such as GPT-3, which is trained on a dataset of over 40 billion words.
References:
· Bengio, Y., Courville, A., & Vincent, P. (2015). Representation learning: A review and new perspectives. IEEE transactions on pattern analysis and machine intelligence, 35(8), 1798-1828.
· Radford, A., Wu, J., Child, R., Luan, D., Amodei, D., & Sutskever, I. (2019). Language models are unsupervised multitask learners. OpenAI Blog. https://openai.com/blog/language-models-are-unsupervised-multitask-lear…
· Vaswani, A., Shazeer, N., Parmar, N., Uszkoreit, J., Jones, L., Gomez, A. N., ... & Polosukhin, I. (2017). Attention is all you need. In Advances in neural information processing systems (pp. 5998-6008).
Even more impressive. Not only does the model generate relevant text and include references inline in each paragraph, even the references themselves are all real academic papers, which seem to make sense in the context of the paragraph. Perhaps this sample would not pass closer inspection. However, it becomes of reasonable quality where you can imagine a paper written in this manner might get a sufficient mark. A recent study even showed how version of GPT-3 trained on GitHub code, named Codex, was able to perform on introductory programming courses better than most students[5]. Not convinced students will try this? There is already a market for solutions aimed specifically at essays that will pass plagiarism checks and promise discretion, such as EasyAILab.com.
So will this be the end of essays, writing in general, and will the AI now take over? Of course, it is not that simple. Is there anything you can do against this? So, far this is still something in development and mitigation might happen in a couple of ways. One approach could be banning or restricting use of this technology, which is at times done for the use of computers or phones in classrooms. But this is difficult for software-based applications. Another approach is to try and mitigate its impact with new forms of ‘plagiarism’-checking. One such checking application is the OpenAI detector developed by HuggingFace, which detects the likelihood a bit of text is generated by an OpenAI based language model. A few small tests seem to show this works quite well. Lastly, you could consider how this new technology will impact existing educational and adapt. Look at this gymnasium school in Germany, that even required the use of GPT-3 in its German lessons and sees how controlled use helps students “learn personal responsibility through the use of AI tools”[7]. I would be curious to hear what your thoughts are when it comes to dealing with this new development.
The impact of this technology could be quite big, with also a lot of interesting potential for improving our ability to engage with texts, write code, or creatively brainstorm. Only with time we will figure out which uses will stay. However, these models are not without their issues. And I shortly want to mention four of these challenges before we end this blogpost: bias, factual correctness, attribution and environmental impact.
Bias
Just like most AI systems, language models struggle with bias. Since the data on which the model is trained will contain certain themes and structures, the model will replicate this. The engineers of ChatGPT try to mitigate these issues by marking certain topics as sensitive. However, as can be seen in the example below, when someone tries to test the model a bit more creatively it becomes easy to see that these biases still exist within the model.
Factual correctness
While the formulation of a generated paragraph or may sound plausible, the actual information conveyed is to be critically checked by the user on correctness. The data used to train the language model includes all sources of information and is not checked on its correctness before training. The way a model such as this works is not by reasoning, but by mimicking its dataset with synonyms and paraphrases. So statistically a sentence might be perfectly sensible for the model that these words and phrases form a sentence, but it does not generate a meaningful abstract relationship. As you can see in the example below. The content often seems sensible because the majority of the training data is text written by humans that is (generally) ‘grounded in the real world’[9].
Attribution
The strength of Large Language Models comes from the data it is trained on. For GPT-3, the model underlying ChatGPT, that seems to be crawled webpages, books, and wikipedia [11]. For models like Githubs Co-pilot, that is their own github repositories full of pieces of code. This raises some challenging issues when it comes to attribution. When humans use resources by others, we are supposed to cite or give attribution and at times are even bound by certain licenses (such as CC-BY). It is only possible to claim full credit for your work if you know and have cited your sources [12].
Already you see responses to this challenge of language models. Image hosting website Getty Images banned AI-generated art works from their service [13]. And one of the most interesting developments is the litigation process against GitHubs CoPilot for violating the licenses of developers [14].
Environmental impact
Lastly an important discussion is to be had about the environmental impact of these technologies. Researchers estimated that training the already quite outdated Transformer (big) model, cited above by ChatGPT, had an impact of 248ton CO2 (the average human emits 5ton CO2 per year) [15]. For the GPT-3 model the environmental impact is still unknown, but the training costs have been estimated in the millions. For SURF the environmental impact of compute infrastructure is an important theme and you can read more about our work on energy aware computing on our website.
Conclusion
These new developments clearly show how language models can and will impact education. It is up to us as an educational sector to deal with it. How exactly is still unknown. As the Onderwijsraad (Dutch Educational Council) notes in their report on intelligent technologies: “Just like when the calculator was introduced in education, consideration must be given to the place of this intelligent technology in education and what this means for learning goals.” [16]. For now I would say, register and try and fill in one of your assignments. Does ChatGPT do a good enough job on your in-class assignments?
Are you curious to learn more? Soon you can expect a follow-up blog (now online) from SURF going more in-depth into the working of natural language processing and large language models (like GPT-3). Interested in the specific impact on assessment? Check out the oncoming webinar on How AI has the potential to disrupt student assessment by our colleagues at JISC (13th of December):
Read more in these other blogposts on SURF Communities (in Dutch):
Do you want to know more about AI in education, or discuss its potential? Reach out to our projectmanager AI in Education bertine.vandeyzen@surf.nl. Want to discuss more about the impact and responsible use, let me know!
References:
[1] Promises of AI in Education (2022) https://www.surf.nl/en/promises-of-ai-in-education
[2] Volkskrant (2022) https://www.volkskrant.nl/nieuws-achtergrond/een-moordscene-a-la-nicci-…
[3] Yes, I left my spelling error in their ;)…
[4] I started with “Can you further specify the section 'How language models work' into how the models are trained and how the models generate the text and include academic references.”, but this ended up as a text with just endnote references and of course a student would have to include in-line references. S
[5] The Robots Are Coming: Exploring the Implications of OpenAI Codex on Introductory Programming (2022) https://dl.acm.org/doi/abs/10.1145/3511861.3511863
[6] ChatGPT – 3hoog https://dub.uu.nl/nl/3hoog/chatgpt
[7] A teacher allows AI tools in exams – here’s what he learned https://the-decoder.com/a-teacher-allows-ai-tools-in-exams-heres-what-h…
[8] Twitter link to image https://mobile.twitter.com/spiantado/status/1599462375887114240
[9] How come GPT can seem so brilliant https://garymarcus.substack.com/p/how-come-gpt-can-seem-so-brilliant
[10] How come GPT can seem so brilliant https://garymarcus.substack.com/p/how-come-gpt-can-seem-so-brilliant
[11] GPT-3 wikipedia page https://en.wikipedia.org/wiki/GPT-3#Training_and_capabilities
[12] The attribution problem with generative AI https://hackingsemantics.xyz/2022/attribution/
[13] Fearing copyright issues, Getty Images bans AI-generated artwork – Ars Technica https://arstechnica.com/information-technology/2022/09/fearing-copyrigh…
[14] Github Copilot litigation https://githubcopilotlitigation.com/
[15] On the Dangers of Stochastic Parrots: Can Language Models Be Too Big? https://dl.acm.org/doi/10.1145/3442188.3445922
[16] Inzet van intelligente technologie – Onderwijsraad https://www.onderwijsraad.nl/publicaties/adviezen/2022/09/28/inzet-van-…
Digitale innovatie voor onderwijs en onderzoek. Nu ben ik binnen SURF bezig… Meer over Duuk Baten
Dit artikel heeft 1 reactie
Als lid van SURF Communities kun je in gesprek gaan met andere leden. Deel jouw eigen ervaringen, vertel iets vanuit je vakgebied of stel vragen.
Is dit artikel ook in het Nederlands beschikbaar? Of: Kan iemand dit artikel met chatGPT even in een mooi Nederlandstalig artikel omzetten? Dan kan ik het namelijk met mijn collega's delen.
1 Praat mee